Techniques for cleaning consumer data in the utilities industry
Introduction
In the utilities industry, data cleansing is a critical process that ensures the accuracy and consistency of consumer data. Data cleansing refers to the process of identifying and correcting errors, inconsistencies, and inaccuracies in data, with the goal of improving data quality. In the utilities industry, data cleansing is particularly important, as it helps to ensure that billing and payment systems, resource planning, and demand forecasting are all based on accurate and reliable data.
Cleaning consumer data in the utilities industry is crucial because it helps to improve the overall customer experience. Inaccurate or incomplete data can lead to billing errors, payment issues, and other problems that can negatively impact customer satisfaction and retention. Additionally, data cleansing can help utilities companies to optimize their operations and resources, reduce costs, and comply with regulatory requirements.
In this blog post, we will explore some of the most effective techniques for cleaning consumer data in the utilities industry. We will discuss data profiling and analysis, data scrubbing and standardization, de-duplication and data matching, data enrichment, and data validation and verification. We will also highlight best practices for data cleansing in the utilities industry and explain the benefits of clean consumer data for utilities companies.
Identify data quality issues in consumer data
To effectively clean consumer data in the utilities industry, it is essential to first identify the various data quality issues that exist. Poor data quality can arise from a variety of factors, including human error, system glitches, and incomplete or outdated data. Understanding the various data quality issues that exist is critical to developing an effective data cleansing strategy.
Some of the most common data quality issues in consumer data include data errors, missing data, and inconsistencies. Data errors can occur when incorrect or incomplete data is entered into a system, such as a customer's name or address. Missing data can arise when important information is not collected or recorded, such as a customer's phone number or email address. Inconsistencies can occur when data is recorded differently across multiple systems or sources, leading to discrepancies in the data.
Poor data quality can have significant impacts on utilities operations. Inaccurate or incomplete data can lead to billing errors, which can result in frustrated customers and increased customer service costs. It can also impact demand forecasting, which can lead to over or under allocation of resources, impacting operational efficiency. Finally, poor data quality can lead to regulatory compliance issues, which can result in fines or legal action against the utility company. It is, therefore, essential to identify and address data quality issues in consumer data.
Techniques for cleaning consumer data
To effectively clean consumer data in the utilities industry, there are several techniques that can be used. These techniques include data profiling and analysis, data scrubbing and standardization, de-duplication and data matching, data enrichment, and data validation and verification. By using these techniques, utilities companies can effectively clean consumer data and ensure that their operations are based on accurate and reliable data.
Data profiling and analysis involves examining the data to identify errors, inconsistencies, and other quality issues. This technique is useful for identifying patterns and trends in the data that can be used to improve data quality.
Data scrubbing and standardization involves correcting errors and inconsistencies in the data, such as formatting issues, misspelled words, and incorrect data fields. This technique ensures that the data is consistent and accurate across all systems.
De-duplication and data matching involves identifying and removing duplicate records in the data. This technique helps to ensure that each customer record is unique and accurate.
Data enrichment involves enhancing the data with additional information, such as demographic data or customer preferences. This technique can help utilities companies to better understand their customers and improve their operations.
Data validation and verification involve checking the accuracy and completeness of the data. This technique ensures that the data is reliable and can be used for decision-making purposes.
Best practices for data cleansing in the utilities industry
To ensure effective data cleansing in the utilities industry, it is important to follow best practices. These practices include establishing clear data quality standards, implementing regular data audits, investing in automated data cleansing solutions, leveraging external data sources, and providing regular employee training on data quality.
Establishing clear data quality standards is crucial to ensure that everyone within the organization understands the importance of data quality and how to maintain it. This includes creating a data quality policy and establishing clear guidelines for data entry, management, and reporting.
Regular data audits are important to identify any data quality issues that may have arisen and address them promptly. Audits can be conducted internally or by an external third-party and should include a review of data quality metrics, such as completeness, accuracy, consistency, and timeliness.
Investing in automated data cleansing solutions can help to streamline the data cleansing process and ensure that it is completed more efficiently. Automated solutions can identify and correct data quality issues quickly and accurately, reducing the time and effort required for manual data cleansing.
Leveraging external data sources, such as customer data from third-party vendors, can help to enrich the existing data and improve its accuracy and completeness. However, it is important to ensure that the external data is reliable and accurate before incorporating it into the existing data.
Finally, providing regular employee training on data quality is important to ensure that everyone within the organization understands the importance of data quality and how to maintain it. Training should include data quality best practices, data entry and management guidelines, and data validation and verification techniques.
The benefits of clean consumer data in the utilities industry
Clean consumer data can have several benefits for utilities companies. These benefits include improved customer satisfaction and retention, increased operational efficiency, better resource planning and demand forecasting, and compliance with regulatory requirements.
Improved customer satisfaction and retention can be achieved through clean consumer data. By having accurate and up-to-date customer data, utilities companies can better understand their customers' needs and preferences, leading to improved customer service and satisfaction. Additionally, clean data can help utilities companies to identify potential customer issues and address them before they become larger problems, leading to increased customer retention.
Clean consumer data can also lead to increased operational efficiency. By eliminating duplicate and inaccurate records, utilities companies can reduce the time and effort required for data entry and management. This can lead to reduced operational costs and improved productivity.
Better resource planning and demand forecasting can be achieved through clean consumer data. Accurate and up-to-date data can help utilities companies to better understand customer usage patterns and demand, allowing them to allocate resources more effectively and efficiently.
Finally, clean consumer data can help utilities companies to comply with regulatory requirements. Many regulatory agencies require utilities companies to maintain accurate and complete customer data, and failure to do so can result in fines or other penalties. By maintaining clean data, utilities companies can ensure that they remain compliant with regulatory requirements and avoid any potential penalties.
Conclusion
In conclusion, data cleansing is an essential process for ensuring that consumer data in the utilities industry is accurate and reliable. By identifying data quality issues, implementing various data cleansing techniques, following best practices, and leveraging the benefits of clean consumer data, utilities companies can improve customer satisfaction and retention, increase operational efficiency, better resource planning and demand forecasting, and comply with regulatory requirements.
It is important to understand that data cleansing is an ongoing process, and utilities companies must continually review and maintain their data quality to ensure that it remains accurate and up-to-date. By investing in automated data cleansing solutions, conducting regular data audits, and providing regular employee training, utilities companies can ensure that their data remains clean and reliable.
In conclusion, clean consumer data is crucial for utilities companies to operate effectively in a competitive and regulated market. By prioritizing data quality and investing in data cleansing efforts, utilities companies can gain a competitive advantage, improve operational efficiency, and enhance customer satisfaction. The benefits of clean consumer data cannot be overstated, and it is important for utilities companies to understand the value of clean data and take action to maintain it.
Share article
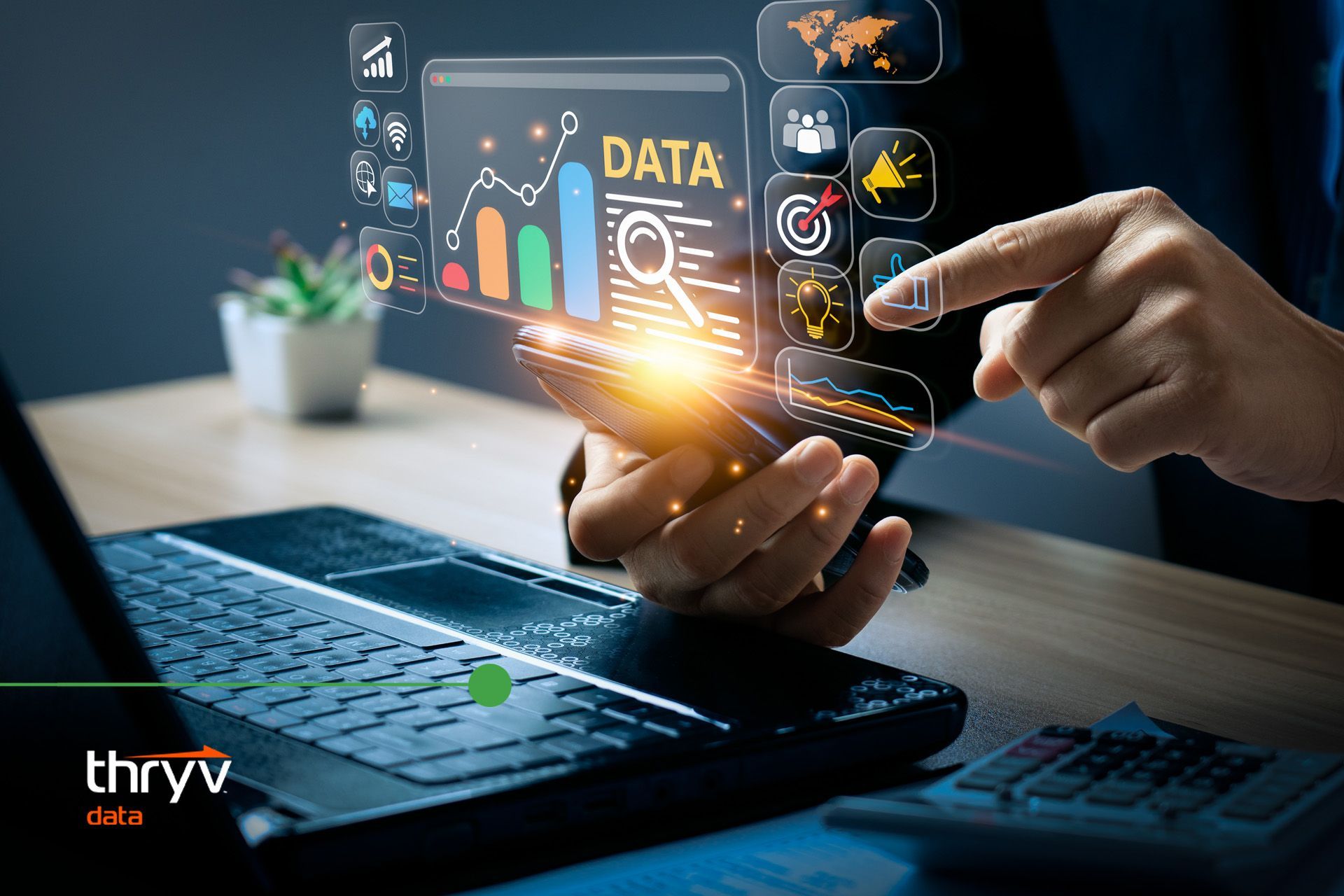
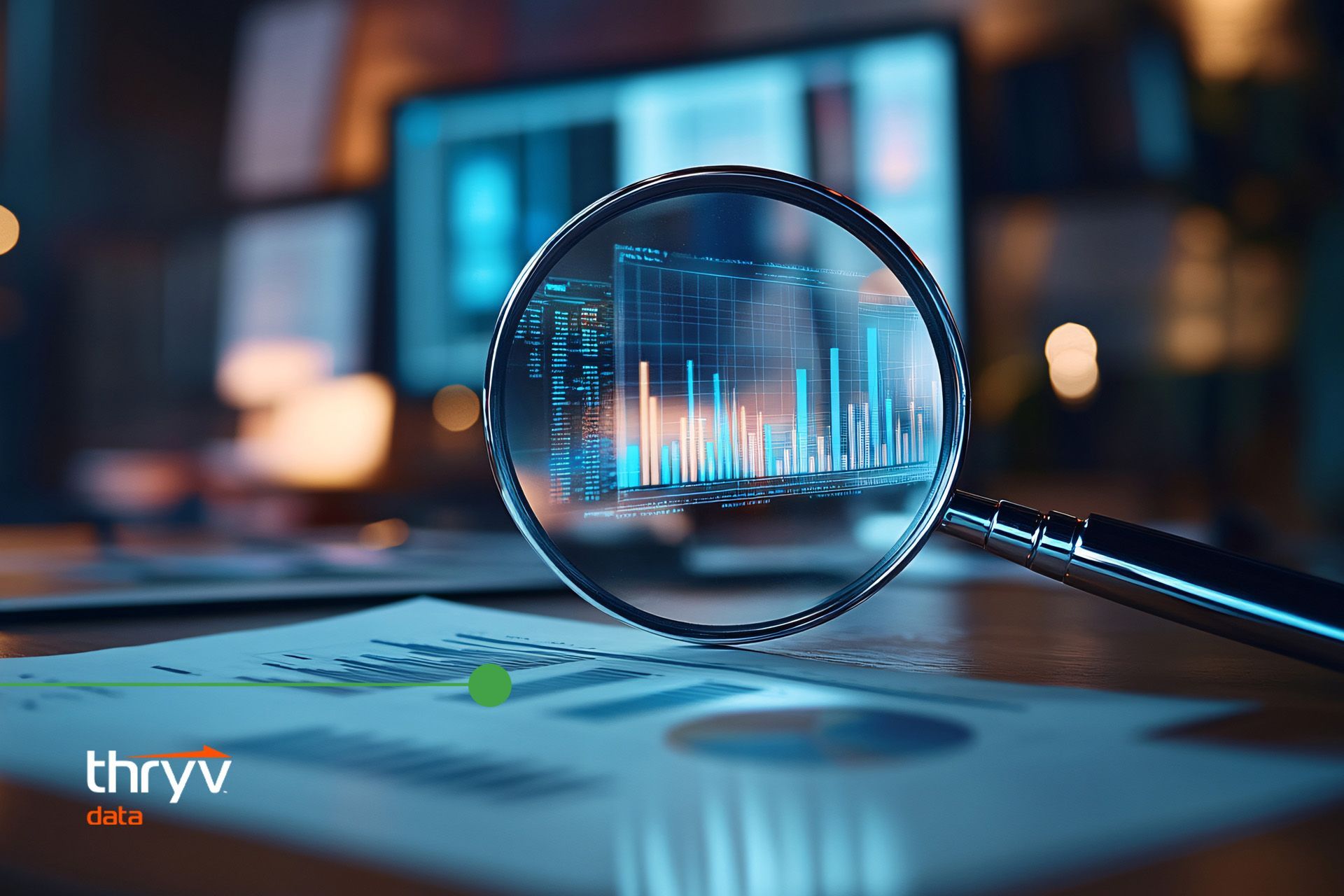
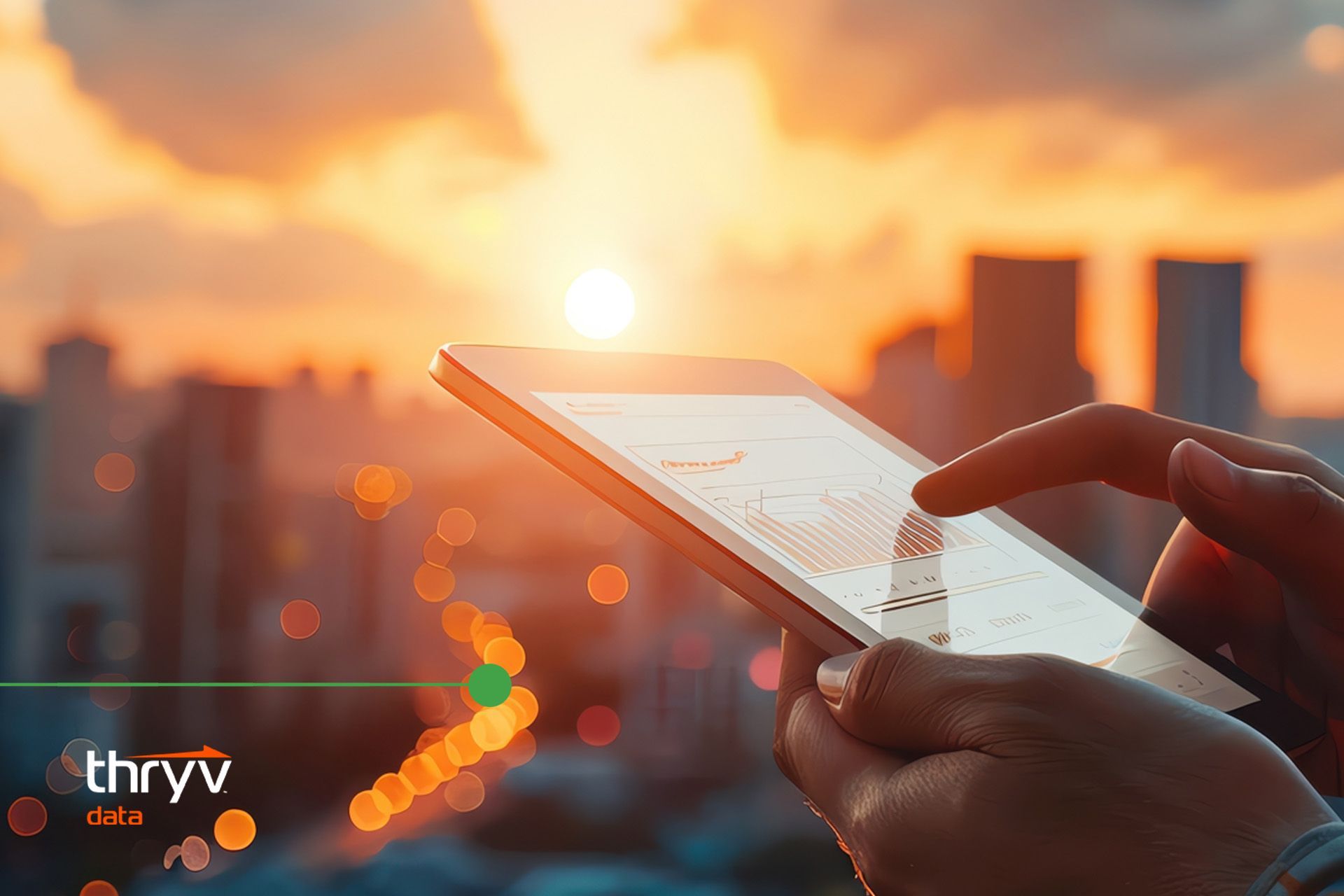
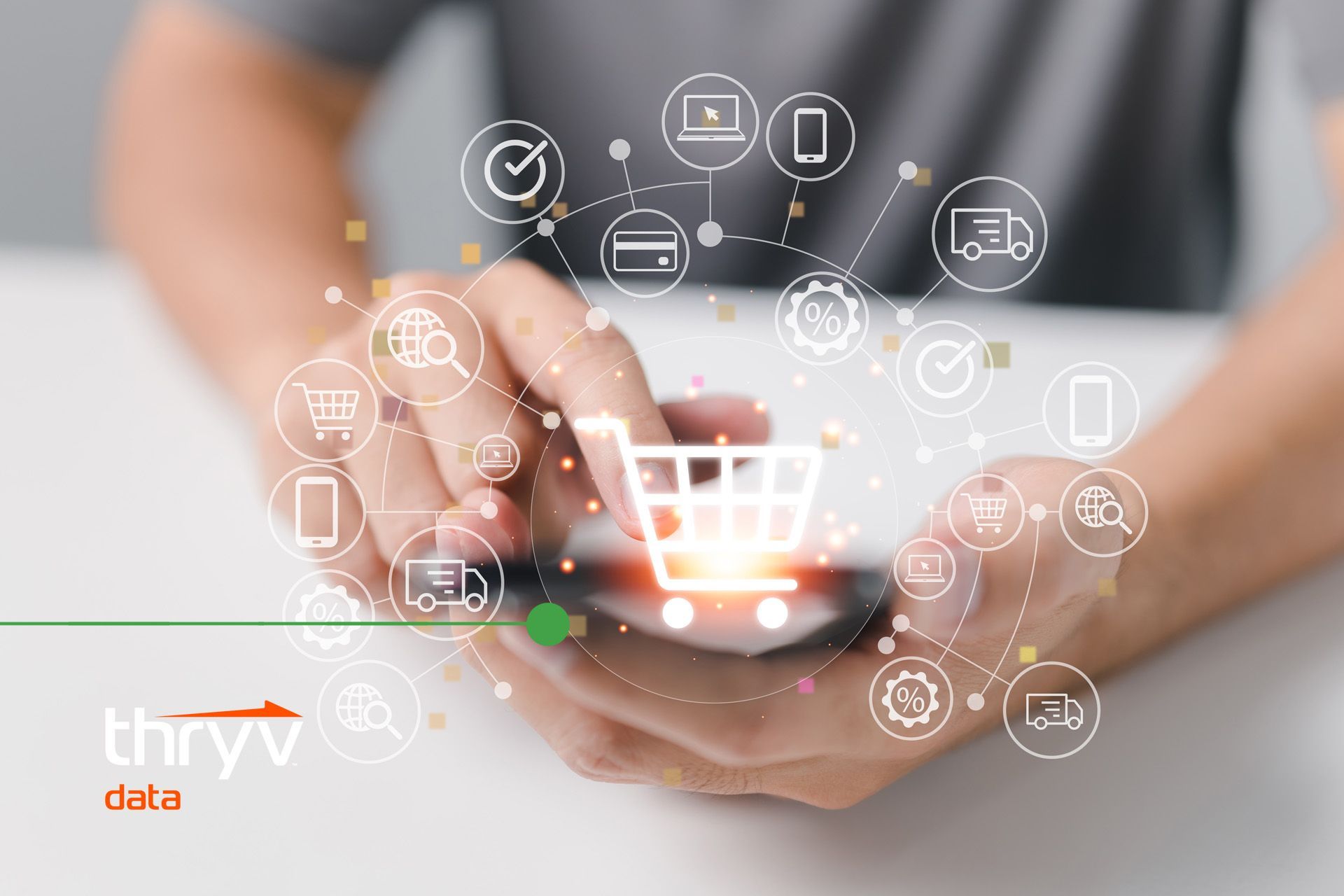
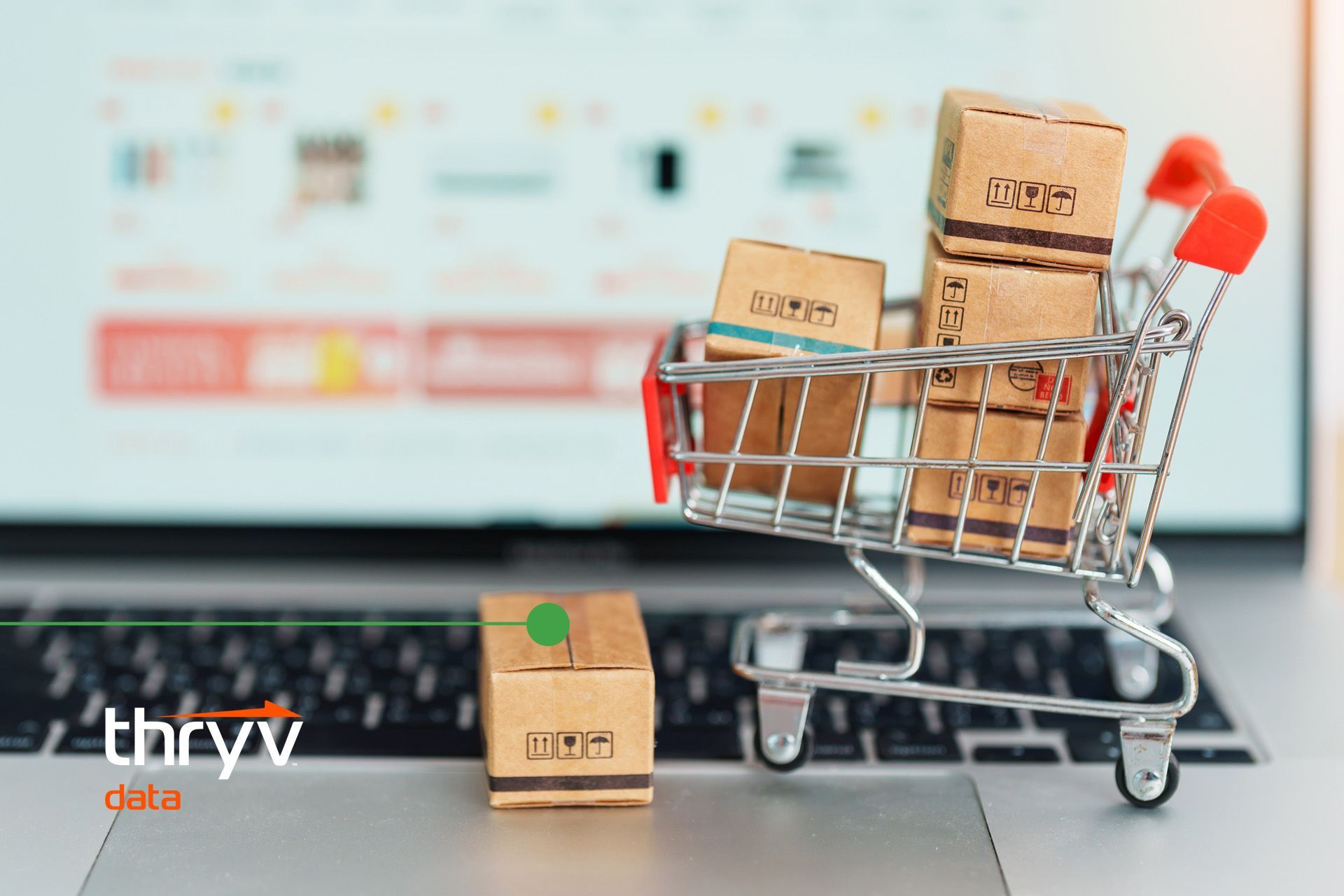
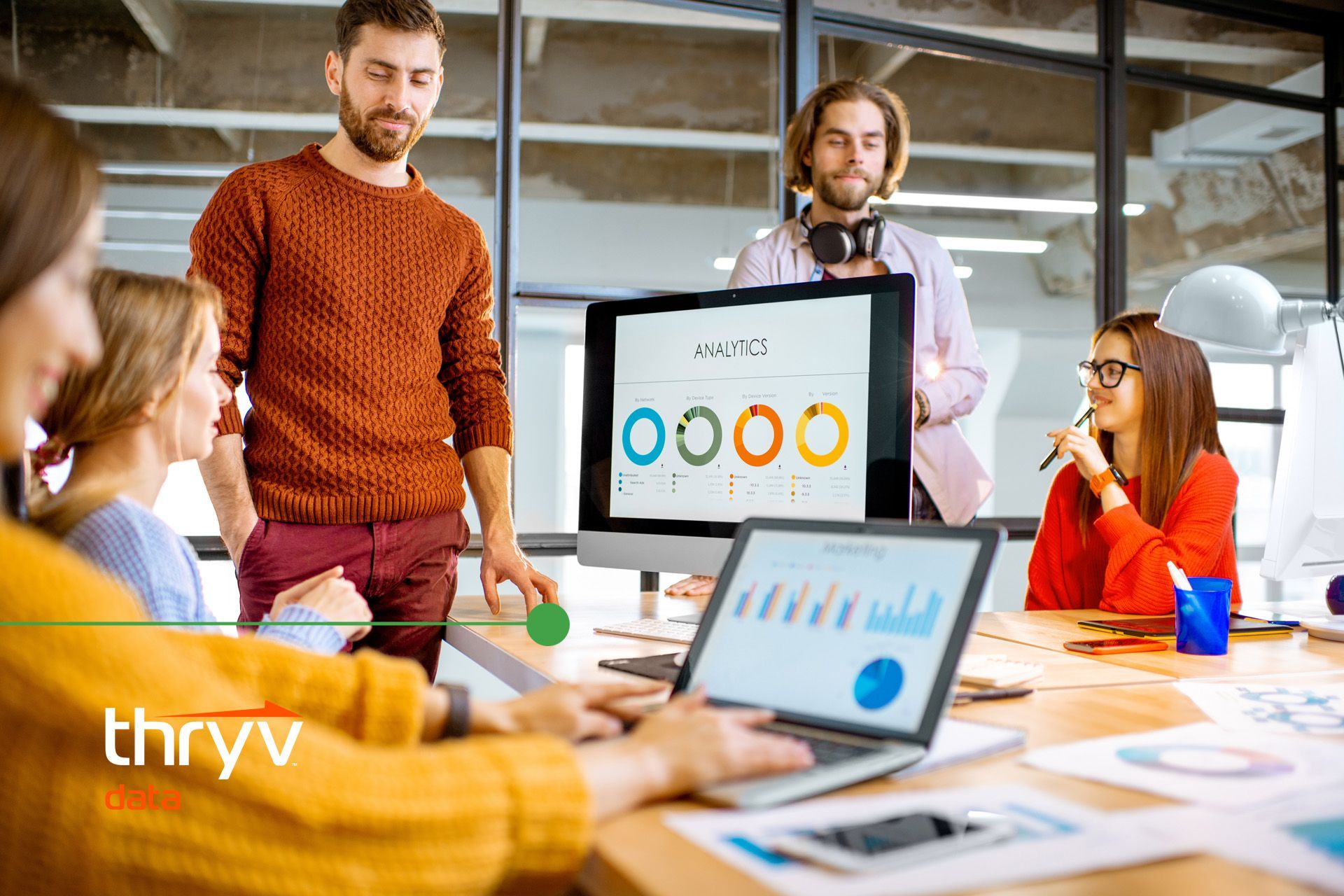
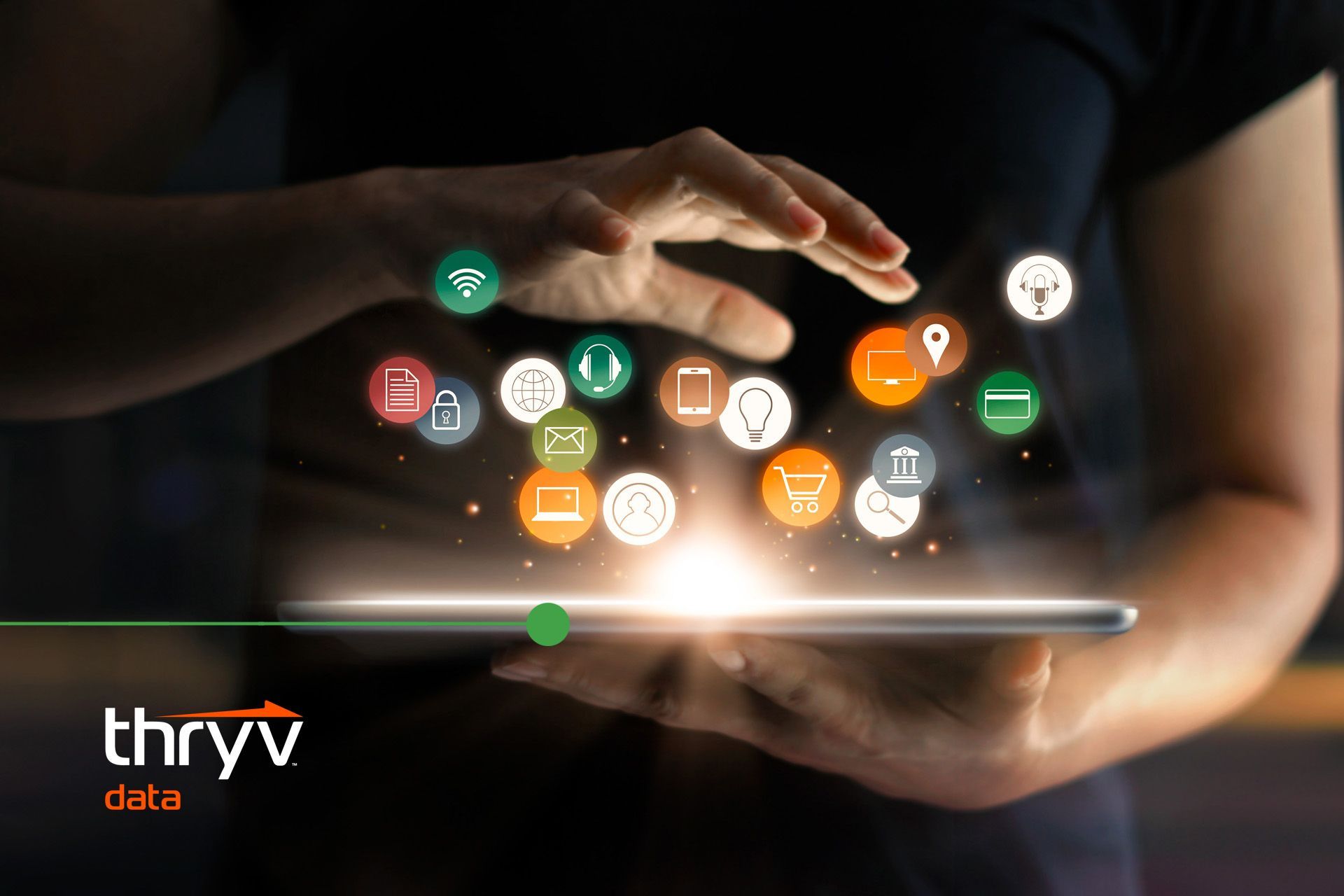
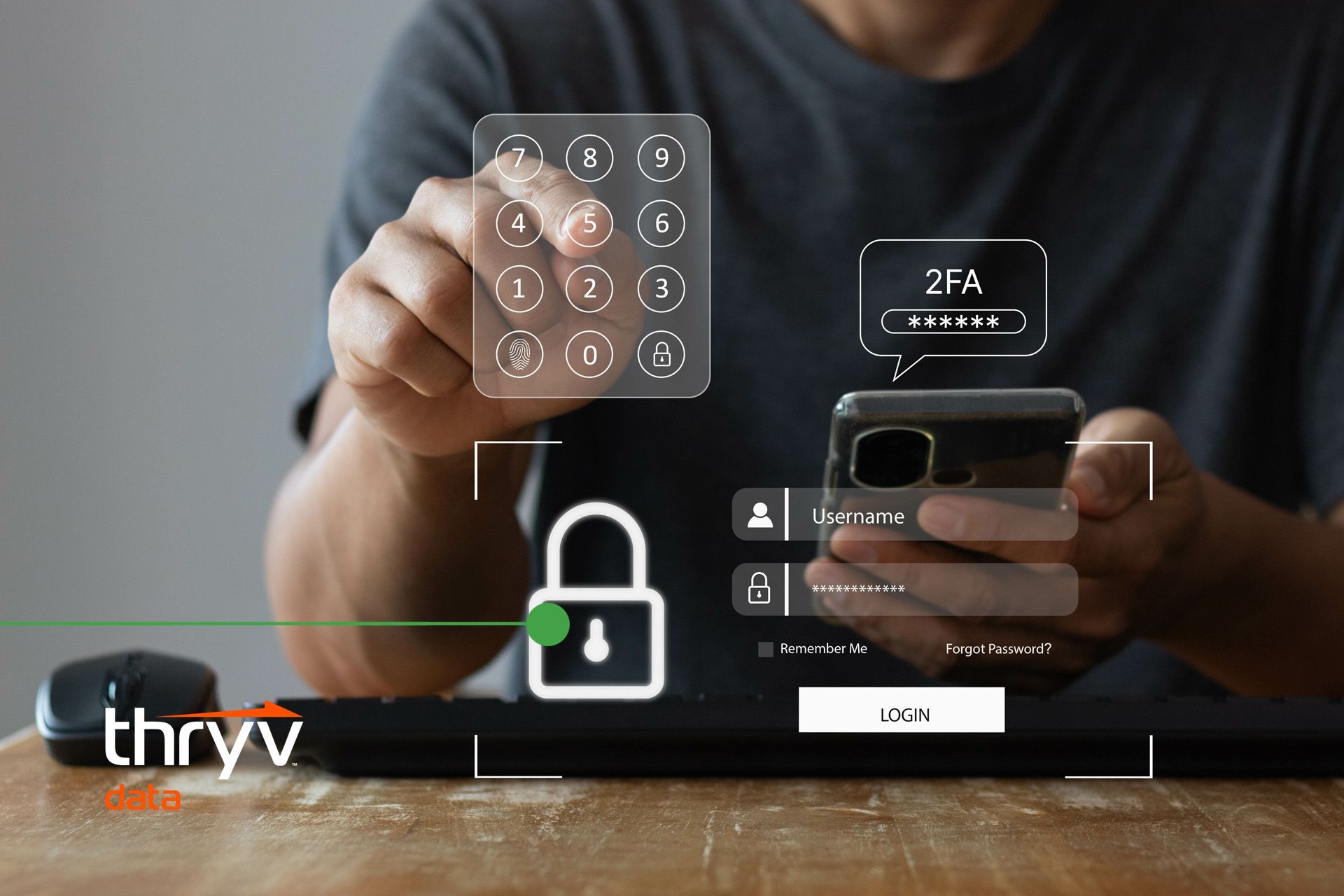
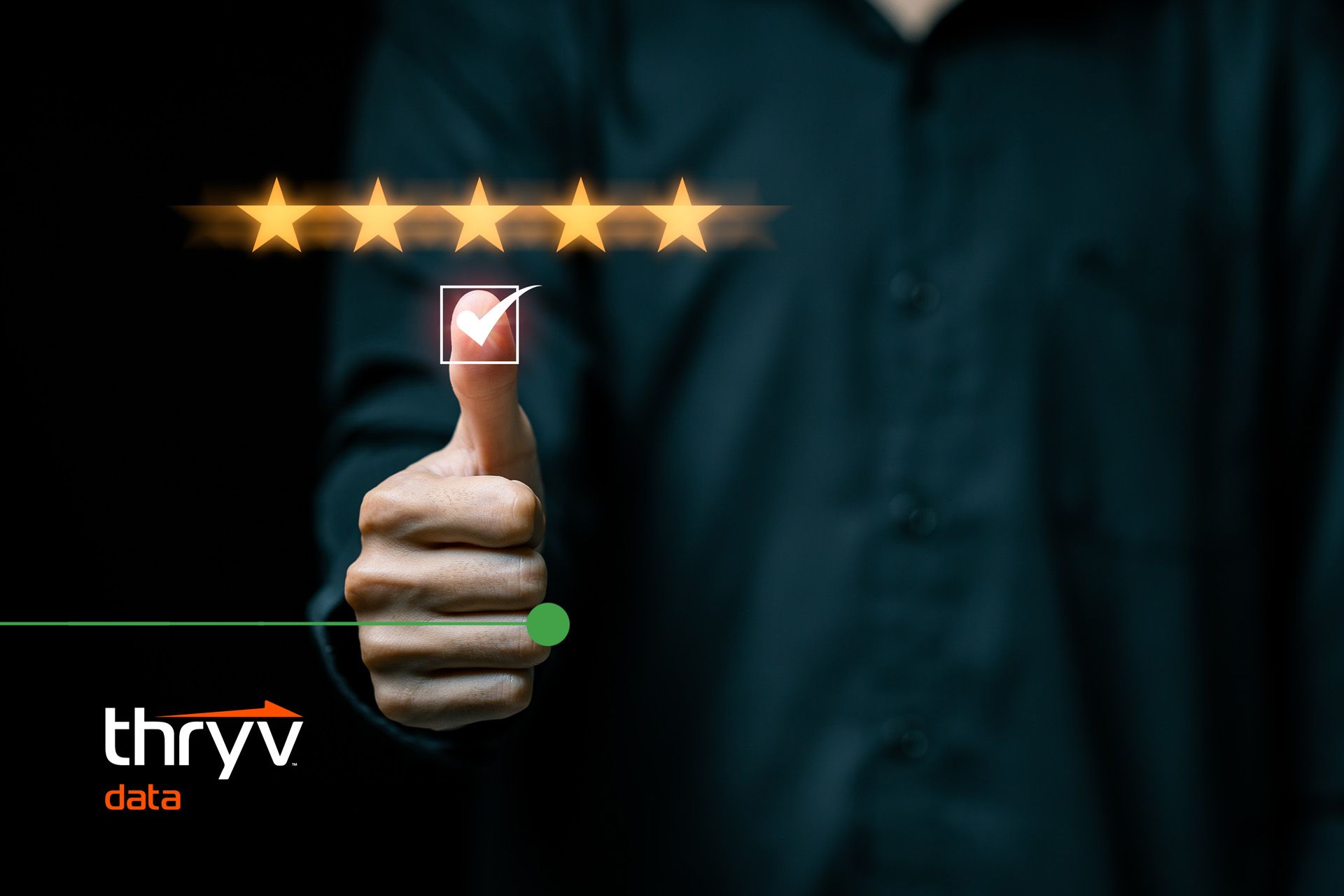